This post originally appeared on the Finn AI blog, which is now part of Glia.
Is your AI experience more positive if your banking chatbot knows your name? This is a question we’re currently examining at Finn AI. Although many people view Artificial Intelligence (AI) as a robotic science, as with any human interaction, there is an emotive component that can be measured. After all, one half of the conversation is driven by a human and it’s the response of the human that we measure.
How We Measure
We use a process called sentiment analysis to determine the outcome of interactions with the [Glia Virtual Assistants] conversational agent. We currently organize outcomes within three basic buckets: Negative, Neutral, and Positive.
As AI data stores increase (and this is occurring at an amazing rate), we will work towards a more refined classification system that will reflect the nuances that typically occur within any human interaction.
In order to assign a sentiment to an interaction, each bank customer utterance is examined by a human being who then assigns a sentiment based on their interpretation of the exchange. We look for positive words (happy, great service, you are the best bot ever), as well as negative and neutral terms.
The final result is an annotated data set that is referenced whenever sentiment is assigned to an interaction. As we add more annotated data, the process of assigning a sentiment will slowly transition to the bot, versus a human.
These bot-ascribed sentiments will be validated by a human and corrected as needed. Each cycle of interaction will allow the bot to increase its accuracy lessening the need for human intervention and providing for a more automated workflow.
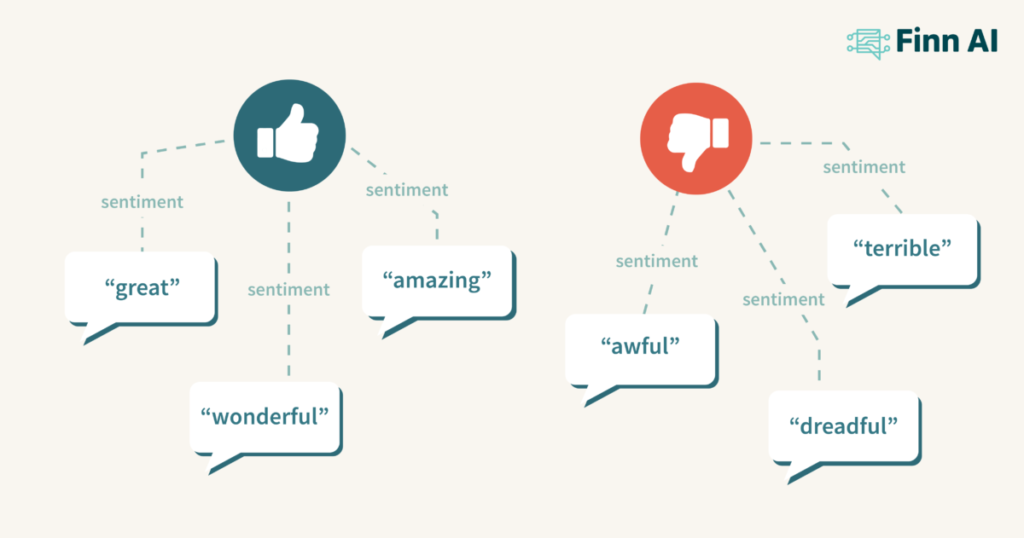
Why Measurement is Important
Every bot interaction includes utterances (what the bank customer says), and intents (what the bank customer wants).
A key benefit of sentiment analysis is the ability to identify the utterances and intents that are triggered prior to a positive or negative sentiment result.
Sentiment can be directed to the bank or to the conversational assistant. A positive response can reinforce the bank’s strategy around the products and services they offer their customers as well as overall satisfaction. Since a key motivator for all of our customers in rolling out Finn AI is to provide a modern and superior experience, a positive result underscores their success in delivering this result.
On the other hand, negative sentiments can flag to the bank that the products and services they present to their customers are not perceived to be helpful or of value. Negative sentiments can also help Finn AI by allowing us to examine and adjust taxonomy design and content which in turn will improve the bank customer experience.
Authenticated vs Unauthenticated Interactions
Finn AI has been deployed publicly with banks across multiple countries and it’s these bank customer interactions that we measure for sentiment. However, it’s important to note that most of these interactions currently occur during unauthenticated sessions. This means the bank customer doesn’t need to log into their accounts to interact with the chatbot.
These chatbot interactions could include looking for answers to general questions, researching and applying for bank products and services, or looking for specific bank information such as branch and ATM locations.
This metered approach to rolling out conversational AI represents best practice and is the way most of our customers choose to deploy. However, from a sentiment analysis perspective, it means the lion’s share of our current data represents interactions where the bot doesn’t know the customer’s name.
It’s important to note that the majority of our customers are actively working on phase two of their Finn AI deployments with capabilities that will require authentication. This will allow us to take sentiment analysis up to the next level.
Why are Authenticated Interactions Better?
When a customer logs into their bank profile while interacting with Finn AI, we’re able to access a richer store of data – and AI loves data! This can include recent transactions, previous research the customer has undertaken, and other activities. Examining this data allows the chatbot to deliver a better result, providing a more curated interaction based upon the profile of the customer.
An authenticated interaction also supports a higher level of engagement which in turn provides the bank customer with a broader experience to which they can assign a sentiment.
Early Results
About 85% of the sentiment analysis we’ve conducted is classified as neutral. The remaining 15% breaks down just about evenly between positive and negative. Of the negative sentiments, about half are directed towards the bank and the other half to the bot.
Next Steps
Right now, since the majority of sessions are classified as neutral, our existing data sets are not balanced. But with so many bank customers interacting with Finn AI, and as more banks roll into phase 2 of their deployment, we will be able to support a more robust sentiment analysis system. This means we can assess chatbot performance and continually innovate to drive optimum customer satisfaction. Most importantly, we can provide our customers with real insight to the performance of their virtual assistant so they can proactively adjust their model to optimize customer satisfaction.
Right now, we’re in the process of proving our theory that customers are happiest when the chatbot knows their name.